Powerful Updates to Novel Computational Imaging Device Featured in Optica
Prof. Lei Tian (ECE, BME) and his team, led by PhD students, Yujia Xue (PhD, ECE, 2022) and Qianwan Yang (PhD student, ECE) published their paper “Deep-learning-augmented Computational Miniature Mesoscope” that describes advances to their Computational Miniature Mesoscope (CM2) project. This paper, published in the prestigious journal Optica, presents the CM2 V2, a more powerful CM2 technology. The most recent developments have been supported by a $2 million R01 grant from the National Institutes of Health.
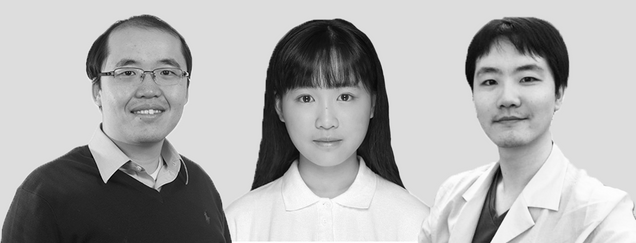
Fluorescence microscopy is key to study biological interactions such as neural activities, gene expression, and molecular interactions. Because the CM2 aims to be applied for studying freely moving animals, researchers who use the device can collect biological data in more native states. This data may help researchers study animal behavior patterns, understand their brain functions, and identify how these functions occur.
Tian is the head of the Computational Imaging Systems Laboratory (CISL) and a faculty affiliate of the Hariri Institute of Computing and the Center for Information and 系统工程. He has been developing the Computational Miniature Mesoscope (CM2) since 2018 along with co-investigators Professor David Boas (BME, ECE, Director of the Neurophotonics Center) and Associate Professor Ian Davison (BIO). They were initially supported by the Dean’s Catalyst award and a BRAIN initiative R21 grant through the project’s developments in 2022 and 2020, respectively.
This CM2 synergistically combines optics and algorithms by using the computational imaging framework to overcome the limitations on conventional fluorescence microscopy. The CM2 prototype combines the merits of both the light field imaging and lenless designs. It attaches a microlens array to an CMOS sensor to capture the sharp high quality images of the sample from different viewpoints. This produced a multiplexed measurement with overlapping views so the 3D fluorescence information could be computationally reconstructed in a single shot.
Tian’s recent paper details that the updated device has an added hybrid emission filter and a miniature collimator to significantly improve the image contrast and light efficiency. The filter suppresses spectral leakage and improves the contrast in the imaging, and the collimator helps collect the light at a broad angle and illuminate the entire mapped area uniformly. This freeform LED collimator, printed and designed by Tian’s team, achieves ~80% light efficiency, three times more than the first version. These updates also achieve a 5x improvement in image contrast and capture a higher quality image with a higher signal to noise ratio.
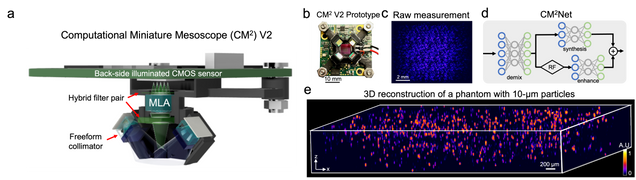
The other crucial aspect of this device is CM2Net, a deep learning model that achieves high-quality 3D reconstruction across a wide FOV and significantly improved axial resolution and reconstruction speed. Deep Learning has emerged as a state of the art technology for solving inverse problems in imaging because it provides fast, high resolution reconstruction and it is time and memory efficient. This deep learning framework uses three modules: View-demixing, view-synthesis, and lightfield refocusing enhancement. View-demixing separates the single measurement into 3×3 non-overlapping views by exploring the differences and rejecting the overlaps in the single shot. View-synthesis finds the depth-dependent disparities and reconstructs the 3D volume, while lightfield refocusing (LFR) enhancement modules use the LFR algorithm to estimate volume, and then enhance, clarify, and match the sample resolution.
This deep learning framework shows potential to provide a high-resolution, 3D reconstruction of the entire mouse cortex and brain vessel networks, and enhances the axial resolution by 8 times that of the traditional model-based reconstruction. The novel 3D reconstruction provides nearly uniform detection performance across the whole field-of-view and is ~1400 times faster and uses ~19 times fewer memory cost than model-based algorithms. This simple and low cost device can be applied in a wide range of large-scale 3D fluorescence imaging and neural recordings.
Learn more about Professor Tian and his students’ work to develop computational imaging methods here.